Personality Models, Management-By Statistic, And Better AI
In which we get out the magic wand and begin our specification of shared language-modifying abuctive reasoning, aka "learning". This will drive architecture and code later.
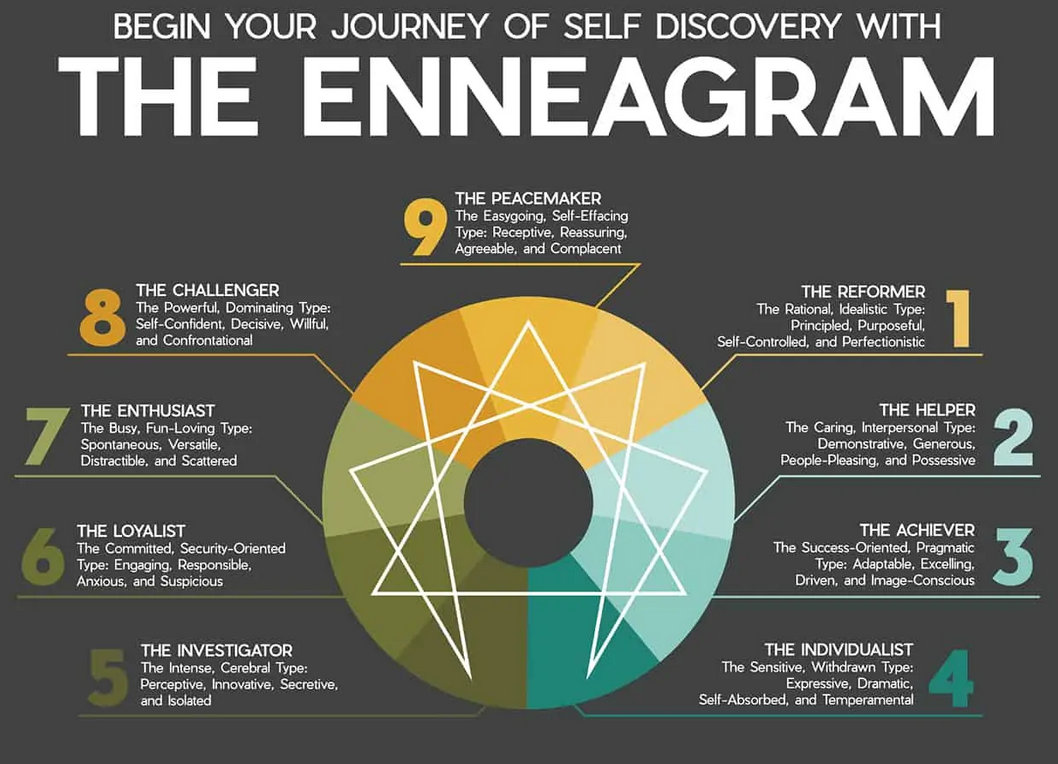
In which we get out the magic wand and begin our specification of shared language-modifying abuctive reasoning, aka "learning". This will drive architecture and code later.